Launched in 2015, Purple has quickly become a leading mattress provider in the US, driven by its unique "Hyper-Elastic Polymer" technology. After several expansions, the business now sells its products through several channels, including retailers and direct to customers.
This combination of a broad product portfolio and various distribution channels generates a high volume of data that Purple’s analysts use to measure the business’ performance and spot new opportunities. However, as the amount of data grew, the company’s legacy data systems struggled to scale accordingly.
“When I joined, we had too many interpretations of data, which led to huge debates in meetings over simple things like dates or gross sales definitions,” explained Matt Meads, Purple’s Senior Director of Analytics
“This became a big problem, especially with changes in our C-suite. New executives questioned the discrepancies in data across different dashboards and systems—where was the right number, Looker or our financial tools like NetSuite and Adaptive?”
These inconsistencies led team members across the business to conduct their analyses using various technologies and systems, creating what Matt described as “a sort of freelance data analytics across the company.” This ad-hoc approach to data delivered results for individual teams in the short term, but as the months passed, the lack of a central source of truth created more and more confusion across the wider organization.
Leadership pushes for reliability
The team recognized that to understand how it was performing, Purple needed a new data system.
“In senior leadership meetings, our CEO would express frustration about inconsistent data and the inability to agree on accurate numbers,” said Matt. “These instances made it clear that we needed to make changes quickly.”
Bryan Kerr, Analytics Engineering Manager, added: “If there’s one single event that kicked off the change, it was when the CEO came to analytics with a request to move BI tools.”
The team soon began exploring options for a new data stack to support their current use cases and allow for future migrations. While attending a manufacturing user group meeting, some data team members noticed many presenters were using dbt. As they began analyzing different architectures, dbt consistently emerged as a best practice due to its simplicity and cost-effectiveness.
“Essentially,” said Bryan, “word of mouth led us to dbt.”
The team soon created a new database for the structured data model within the business, shifting their data ingestion from Matillion & Fivetran to AWS Glue & Fivetran while still channeling data into Snowflake. They also integrated dbt Cloud as the modeling layer, utilizing Looker for data visualization and Atlan for data governance.
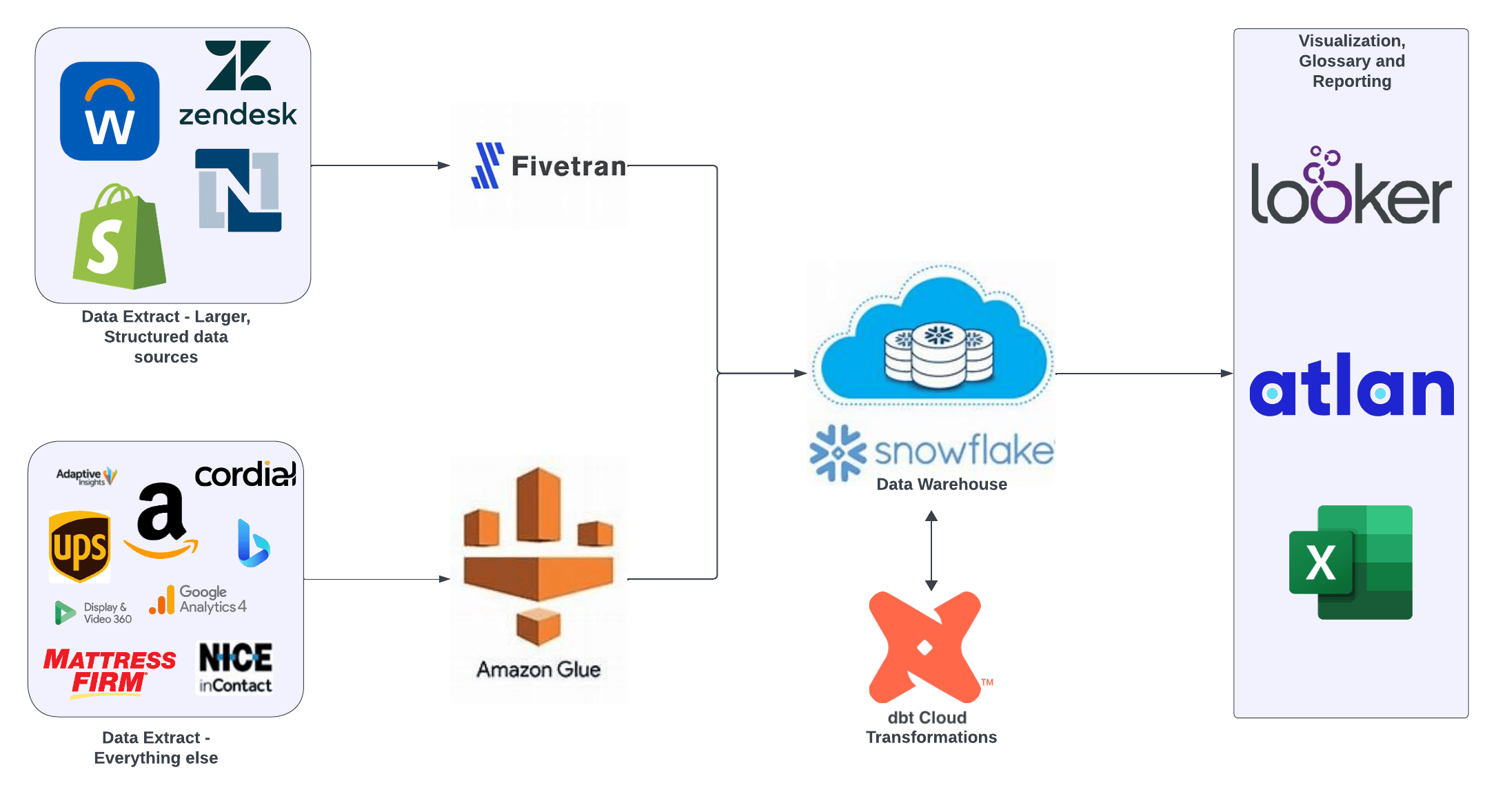
Modularizing complex business logic
Once set up, the new tools quickly delivered results. The team soon found the new setup simplified handling the complex business logic underpinning Purple’s core sales model.
“Previously, any modifications we made to the model would inevitably disrupt other elements, so we were in constant firefighting mode,” explained Matt. “With dbt, we've established a development and QA process before pushing changes to production. Now, we have a more structured, safer approach to making changes.”
This governed approach reduced the time spent on bug-hunting and maintenance while improving trust in the data across the business.
“The value of a good data team is reflected in the quality of data provided,” noted Bryan. “You can’t put a price on trustworthy data.”
Rather than having to hunt through multiple definitions for the same metric, decision-makers can now have confidence in the data they work with—allowing them to act more decisively and in alignment with the data team.
Minimizing on-Call support
Another major success of Purple’s new architecture was reducing the time and energy devoted by the data team to support duties.
The previous ad-hoc approach to deployment meant the data team would run into issues every single day. The on-call staff always had to be ready to firefight issues like broken dashboards and data inconsistencies.
With the new, safer development process, the team now deploys changes twice a week, only needing to monitor for problems for two hours immediately following deployment.
“There are always humans in the process, so there will always be a need for support,” said Bryan. “But with dbt’s monitoring and Atlan’s ability to detect downstream issues, our support demands have reduced significantly. They’ve gone from being a constant, 24/7 requirement to just a few hours a week.”
Matt explained that dbt’s proactive monitoring and testing abilities have been “game-changers.”
“We can often address issues before they even result in tickets,” he continued. “The data source freshness tests are especially helpful, allowing us to identify upstream problems early. We've also implemented various built-in and custom tests to enhance our monitoring.”
dbt has reduced the time the data team spends on support rather than development and improved morale by virtually eliminating one of the most stressful aspects of the data team’s jobs: the Monday morning support rush.
“Monday mornings were just the worst,” winced Matt. “If we let somebody push to Snowflake on a Friday, without fail, on Monday morning, something was broken. Every Monday was dedicated to support for at least two or three people.”
“The transition has made Mondays far less stressful,” added Bryan. “It’s almost eliminated Sunday night stress!”
Optimizing costs as data expands
As the volume and complexity of data models increase, it tends to drive up computation costs. However, despite the implementation of dbt coinciding with a significant corporate acquisition by Purple—and the added strain associated with managing data from the new business—the company found that its costs have remained stable.
Some of this stability is thanks to the data team’s careful management, but Matt also attributes much of the cost management success to the increased efficiency of the new system.
“Moving our business logic from LookML to Snowflake and utilizing dbt Cloud for modeling has significantly reduced our Looker warehouse compute costs,” he explained.
“Our latest consumption reports show stable computing levels, which is notable given the additional systems integrated since adding IntelliBed (the acquired company). Overall, we believe our tools and actions are effectively managing our compute costs.”
Managing a major data re-architecture
With the new data stack in place, the data team finally emerged from firefighting mode and began planning for the future. These plans have combined into a major re-architecture effort driven by dbt.
The project's primary goal is to supply consistent and reliable data across all departments.
“We need to ensure that our data is uniform and trustworthy across various analytics teams. This project aims to build confidence in our data, enabling better business decisions and reducing skepticism and deflection at the top level.”
Bryan added: “dbt offers simplicity. We want to efficiently handle data requests, aiming for a turnaround of a few days rather than weeks or months. It's about increasing efficiency and response time for our data needs.”
The extensive project is ongoing, with the team’s current focus on retiring Matillion. The team is transitioning Python scripts and queries from Matillion to AWS Glue for source data and rewriting transformations in dbt for data marts and similar tasks.
“With Matillion, it was difficult to separate what was a source transformation versus what was a transformation getting used in Looker, he said. “With the project structure we have in place now in dbt, it's all very clear. And we’re working on extending that clarity across the company.”